New study shows that the ketogenic diet significantly increases the risk of heart attack and stroke. Social media influencers in shambles. pic.x.com/3dnKAyUam4
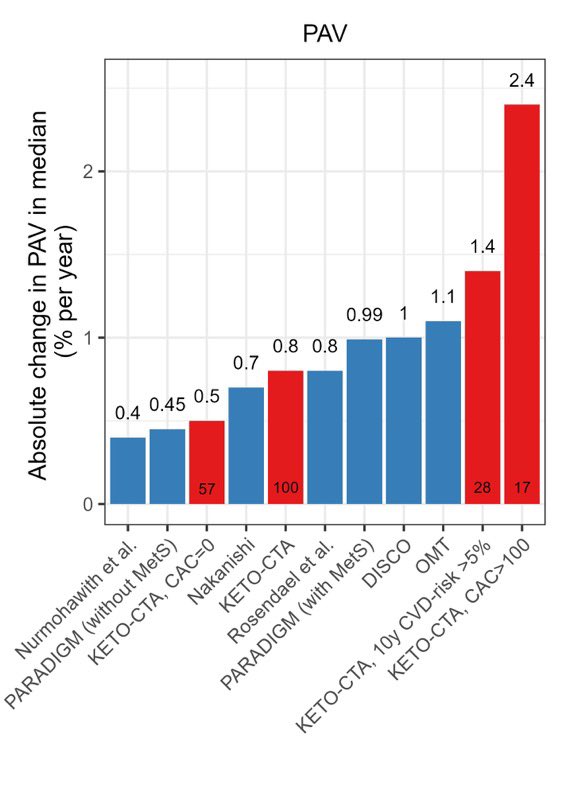
DSPy's biggest strength is also the reason it can admittedly be hard to wrap your head around it. It's basically say: LLMs & their methods will continue to improve but not equally in every axis, so: - What's the smallest set of fundamental abstractions that allow you to build x.com/DSPyOSS/status…
agenetic interfaces will eat chat interfaces. that doesn’t mean there’s not an input filed to type instructions in... but the job of talking to AI models will be mostly done by AI models. pic.x.com/Hdw9TLeOER
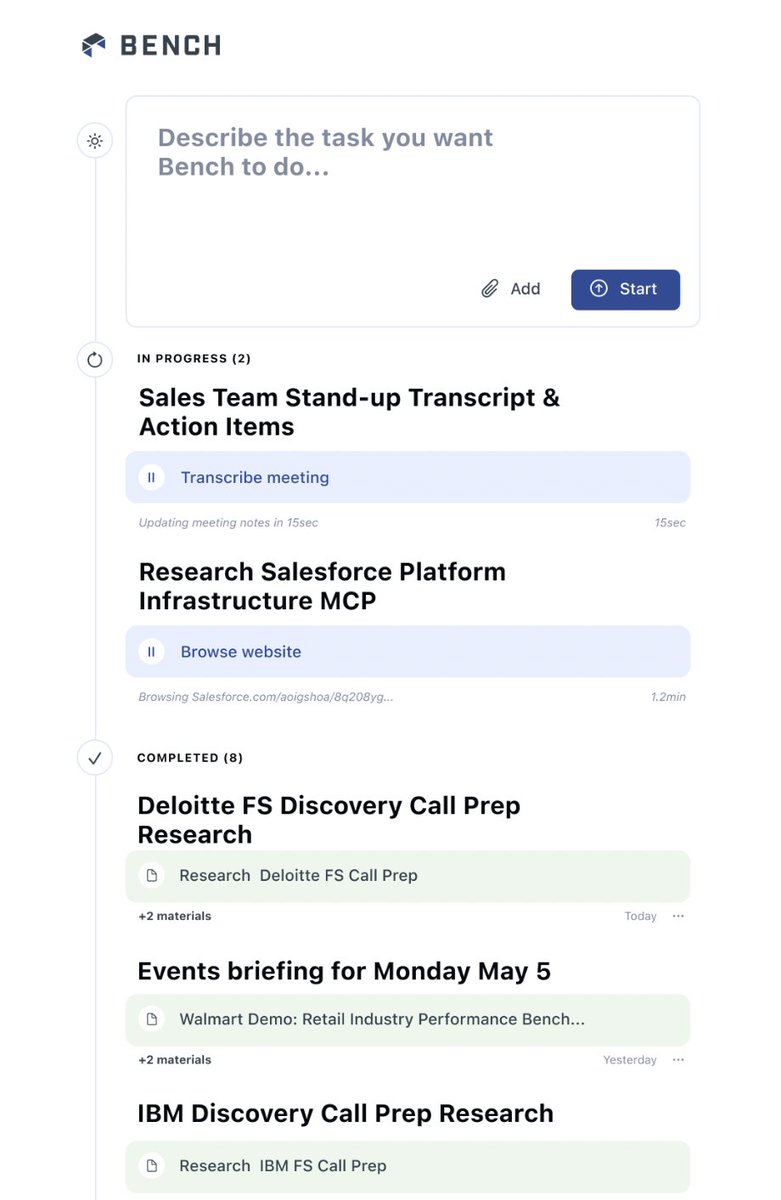
I am excited to announce my new business model interfacer.co Your all-in-one design partner for startups that value speed, clarity, and good taste. faster, together! wish me luck pic.x.com/BjJir9y05j
ADHD is like you wake up every day and have to rebuild the cultural fabric of an entire civilization in your mind from scratch in order to figure out whether you're supposed to, like, do the dishes or whatever
losing my mind over this group of 30 friends who not only actually built a village together but also co-own a factory in this idyllic area? pic.x.com/RtYQneACYH
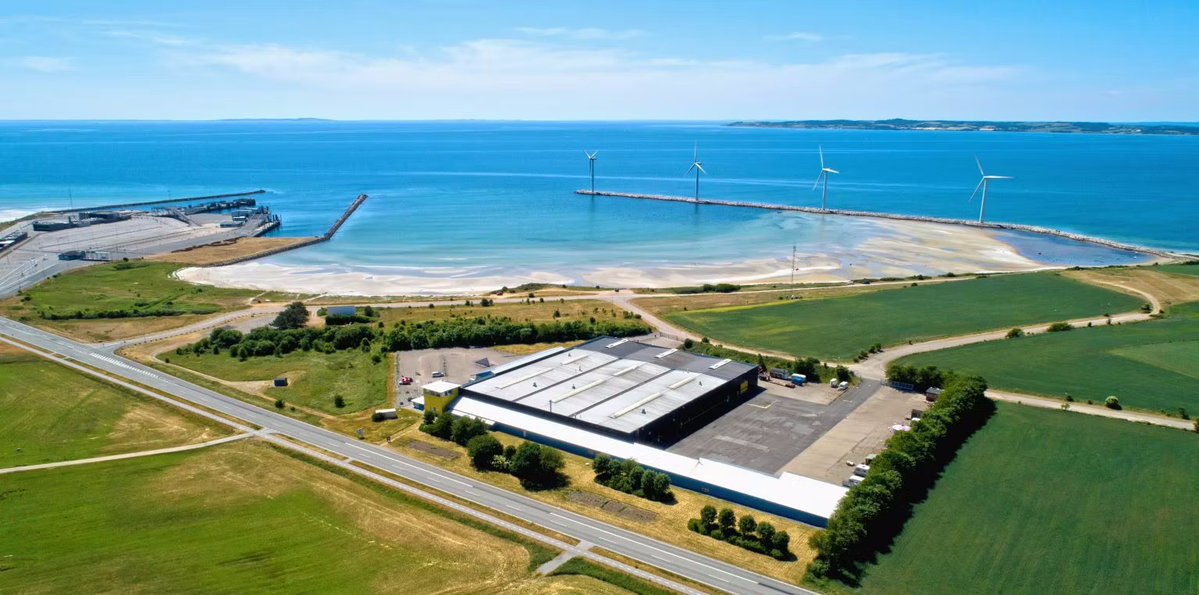
How to know if someone is emotionally mature: pic.x.com/RqkYoK0Ch9
low key obsessed with @inertiajs + Rails + React. I built this Notion like UI in a day (and I don't know React or Typescript). Not even sure if I'm doing it right (am I supposed to redirect to the parent page if I'm just updating a component??) but it just works ™️ pic.x.com/iJvMzln6Eb
Kids should not be so depressed about school that you can infer the school calendar based on suicide attempts. pic.x.com/IGBMEAOgws
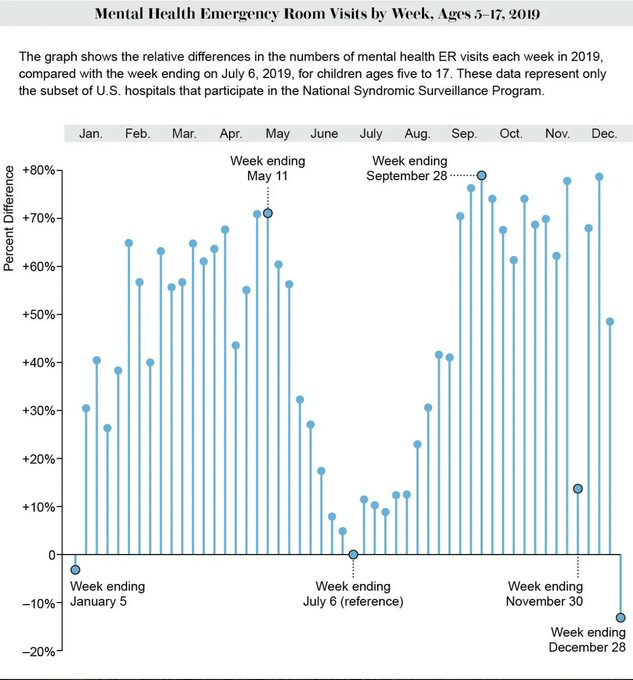
Never going to stop posting these two Many lives will be saved thanks to them pic.x.com/ACBPOxkc8o x.com/WillManidis/st…
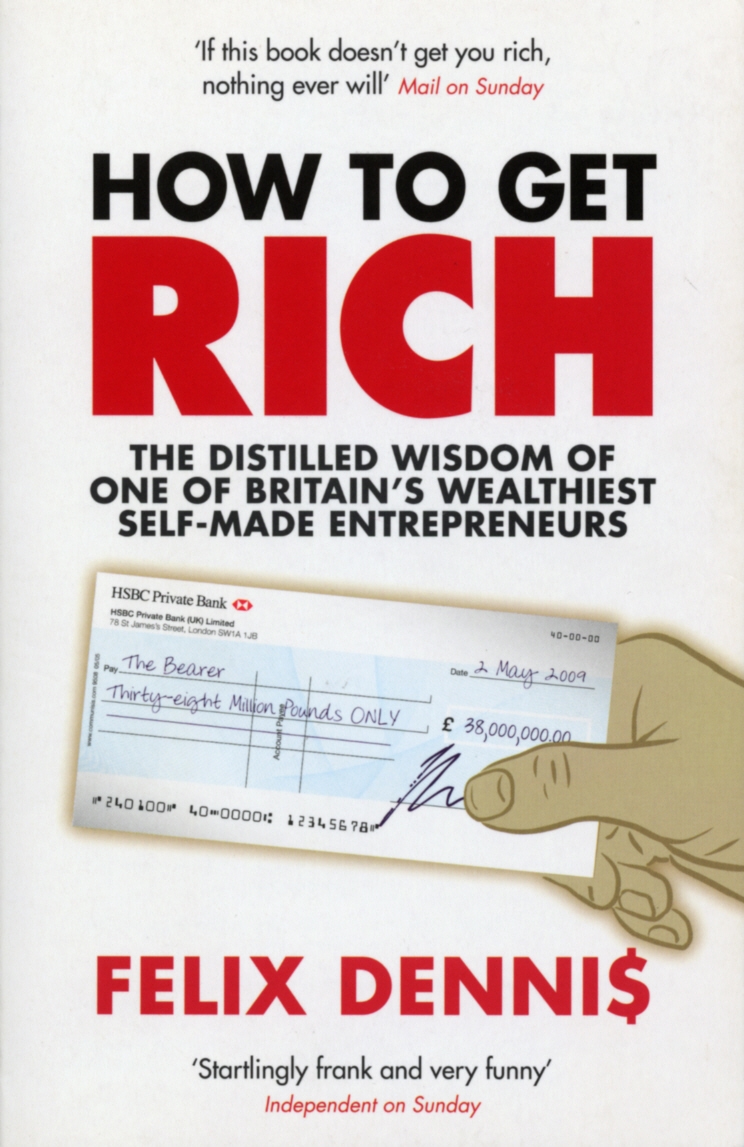
Who is building an web app or website that is going back to basics? No meta JS frameworks, just HTML over the wire.
these 4 fonts are fresh ↓ download below pic.x.com/1HbTSUkMMM
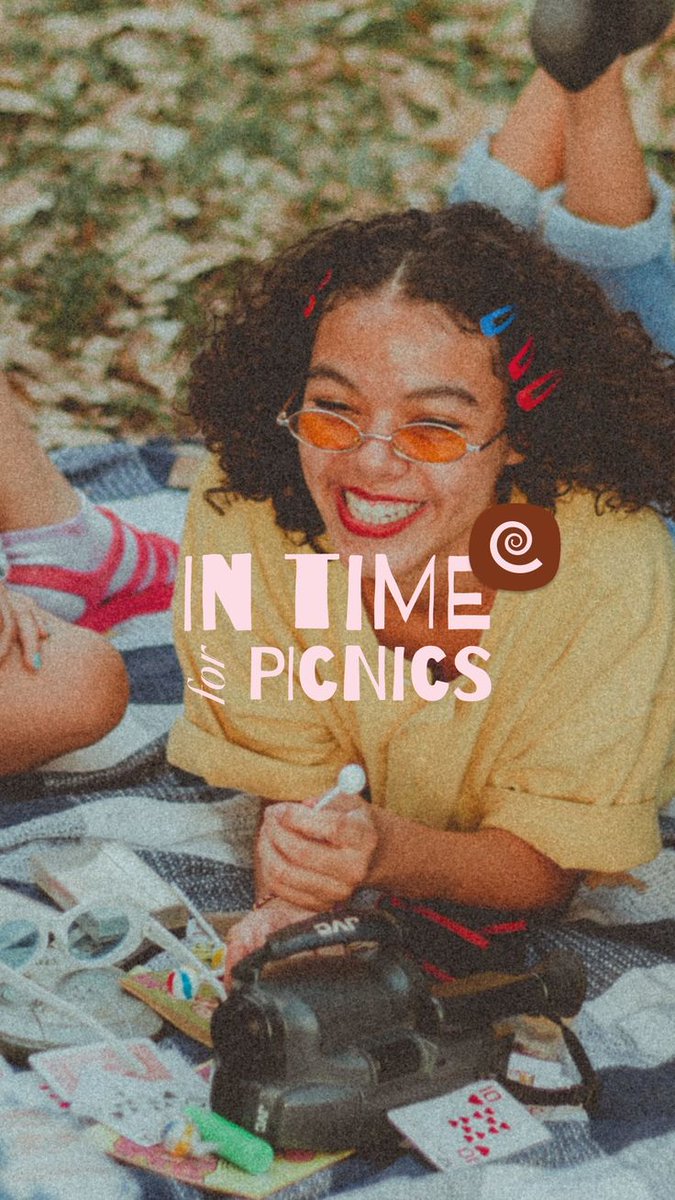
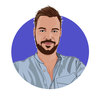
I like picnic club font
Prediction: Trump and Elon will have a significant falling out before the 4-year term is over. There can only be one main character at a time.
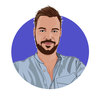
think there’s a very good chance of this happening
Grandpa is really creative🤩😍 pic.x.com/pHhrulGrXN
Weak parents complain in front of their kids... "I hate doing the dishes" "I don’t want to go to work" "Why does this always happen to me..." Victim mindset impacts your child more than you think Here's what to do instead 🧵 pic.x.com/fFIQ6GPGZT
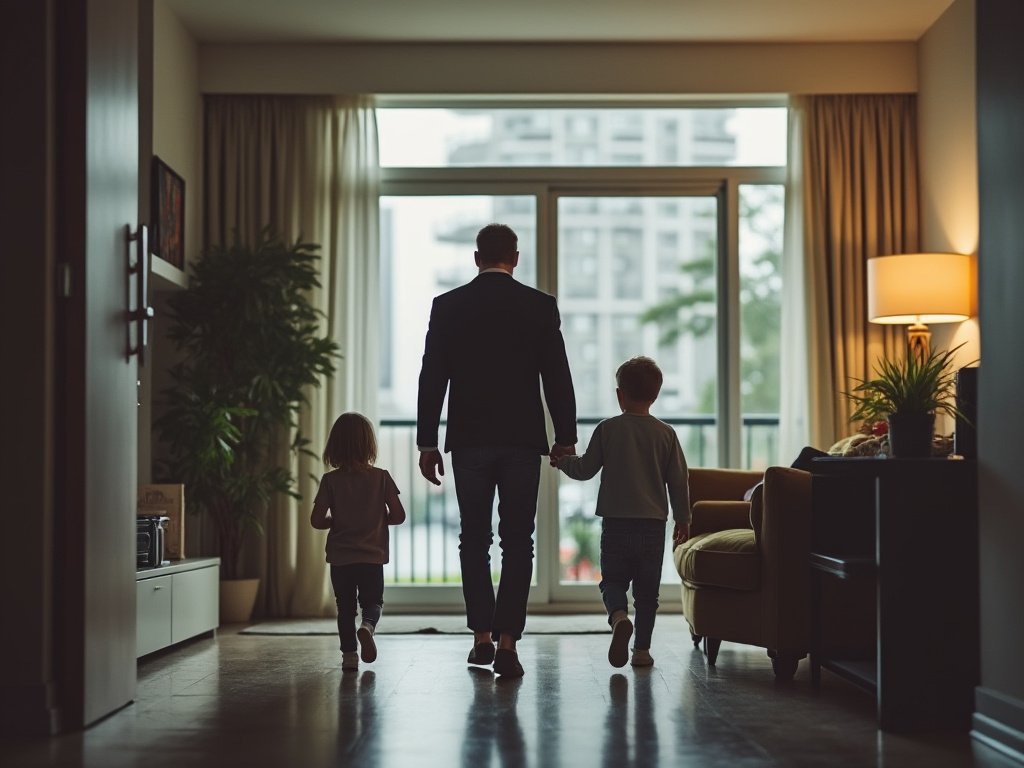
Does your family games night need an upgrade? This family shows some great examples of how to make games night fun, engaging & challenging Kids dont always want to just play Monopoly They want to run, jump, roll around & throw stuff So create activities that tap into this.… x.com/i/web/status/1… pic.x.com/LNmCweVKCC
Here’s a thread rehashing the psychotech and physicaltech that’s helped me the most over the two years, a period in which my wellbeing has improved dramatically: x.com/nosilverv/stat…
A parent's energy sets the tone for everyone in the home Negativity & low energy behaviour will result in kids being negative with low energy If you want your kids to be happy, enthusiastic & positive, Then you need to lead the charge! Your kids feed off your energy Blast… x.com/i/web/status/1… pic.x.com/VT4v3El2VG
High protein diet AFTER fat loss prevents weight regain by fighting microbiome change "refeeding a high-protein diet after short-term dietary restriction attenuates intestinal lipid absorption and represses fat accumulation by preventing Lactobacillus growth." pic.x.com/qaOFThacwg
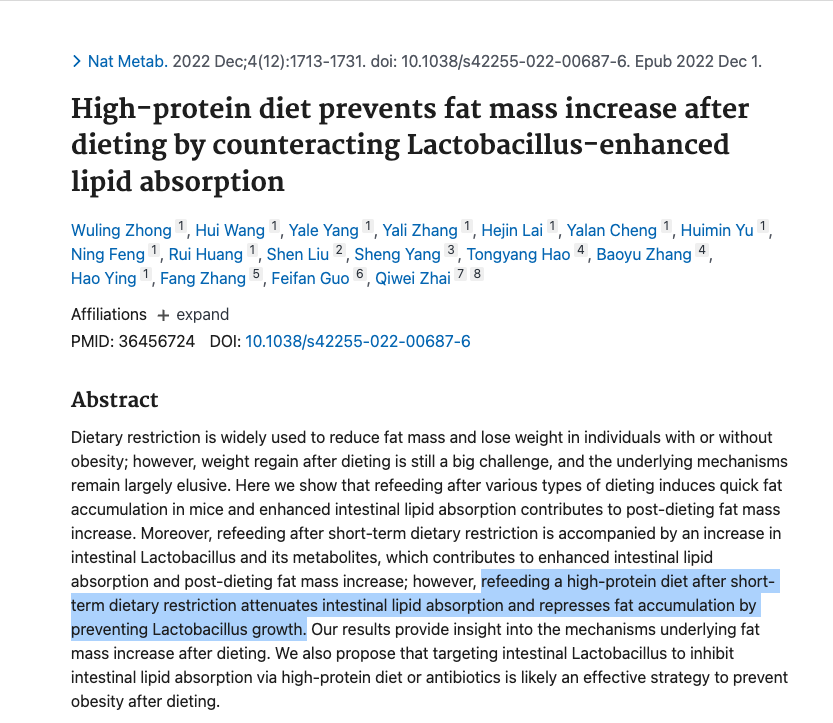
amphetamines make me feel like it’s possible to sit on the porch and watch my kid ride her bike for 5 minutes without getting bored amphetamines help me remember to stay on the couch and rest when I’m sick instead of getting up to do whatever chore just occurred to me pic.x.com/Sb5Z2TfTg5

Today I relearned about the `will-change` CSS property. It helped me go from dozens of dropped frames to nearly zero! It even helps with pure-CSS animations, like this one: pic.x.com/EiNoft2YE6
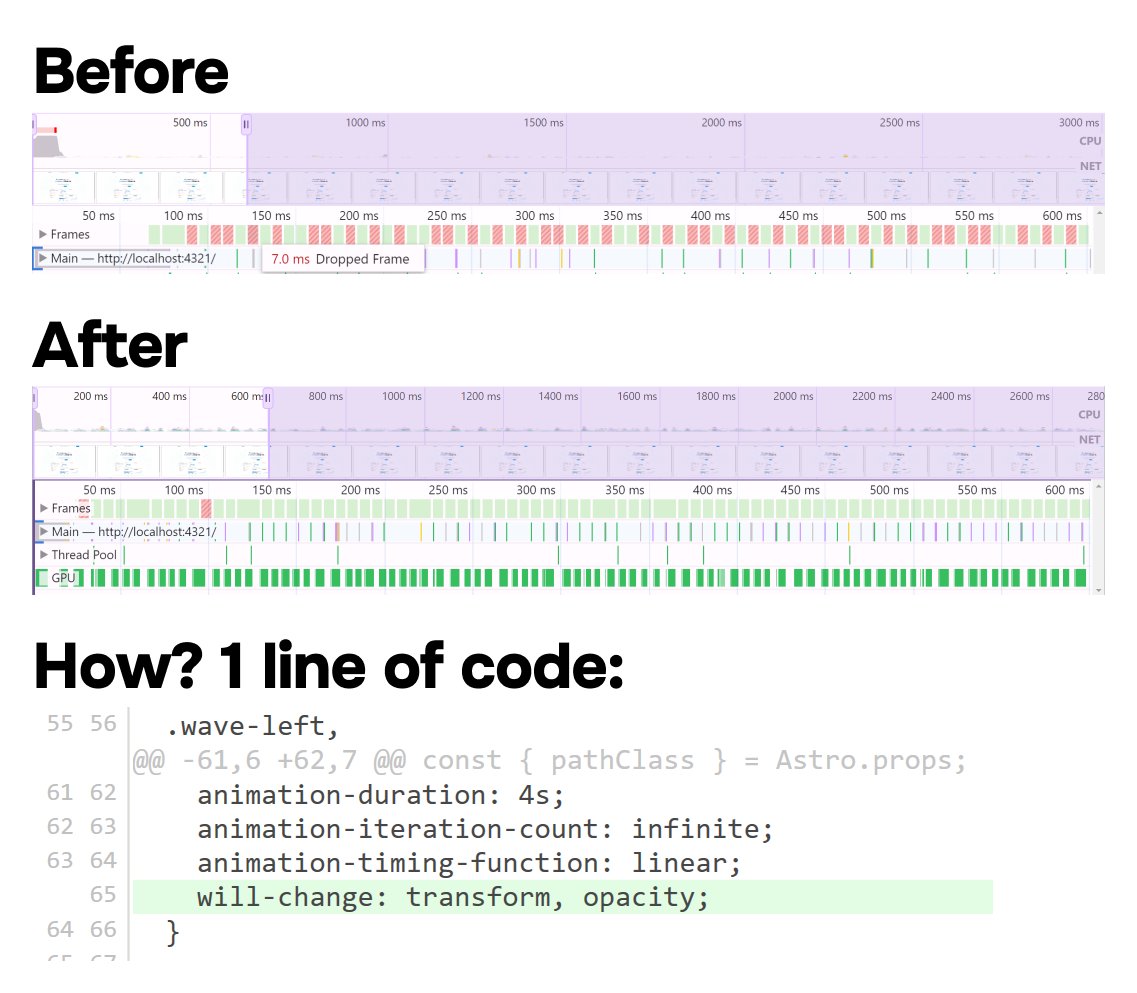
Lung cancer. Given 3 months to live and still alive and well 3 years later. Treated at a clinic in Mexico with the Universal Antidote. pic.x.com/9SvK55O8RM
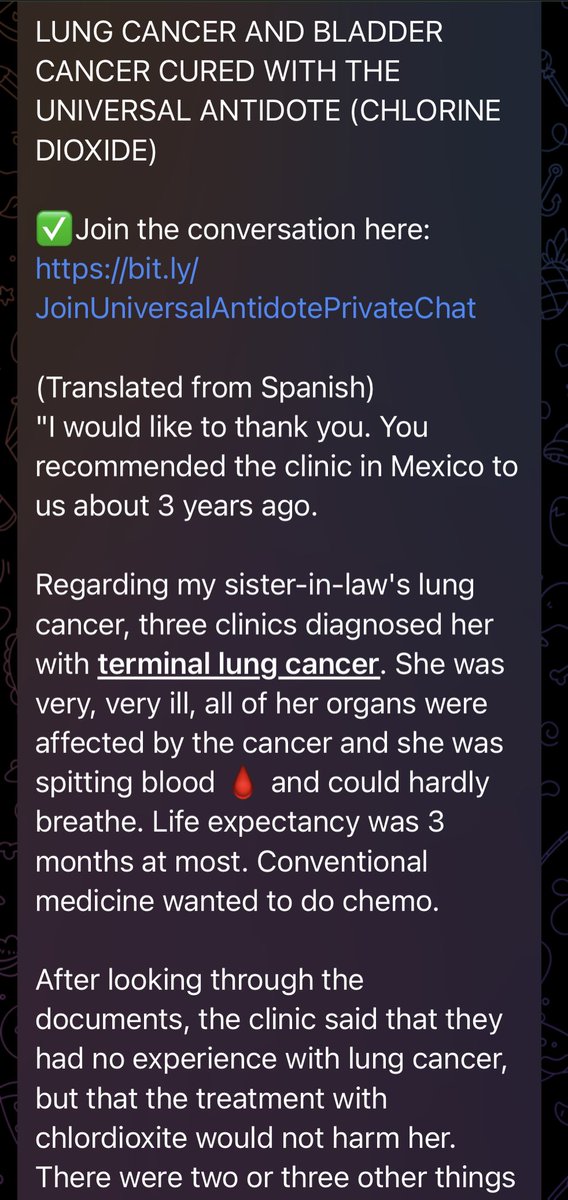
Once we became profitable @typesense, my conversations with VCs shifted. I’d tell them that capital is no longer a constraint for us. So are there any other non-monetary benefits they bring to the table? Till date I haven’t heard a convincing answer Some of the stuff I heard:
my new spot for landing page inspiration → purelanding.page pic.x.com/L4QxDDViNG
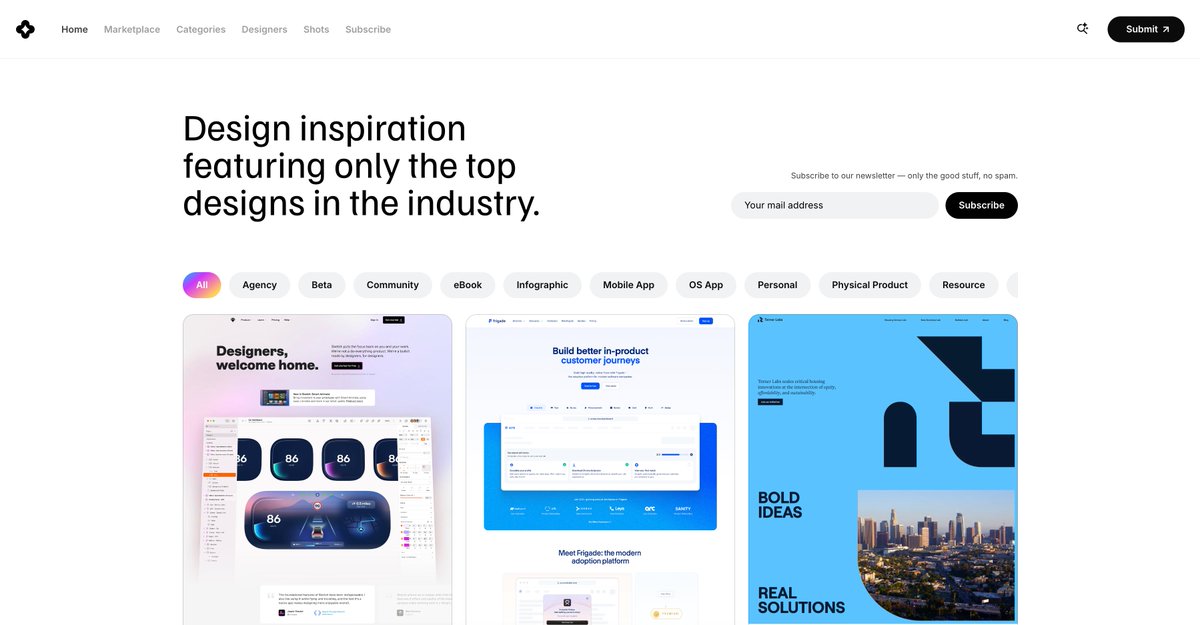
The easiest way to make $10k/mo on TikTok shop is to steal content Literally just find winning viral content on kalodata, download the script, convert it to Spanish using DeepL translate, then make an audio talking clip using elevenlabs voice synthesis. If not now, when? pic.x.com/cSbsrTCZsi
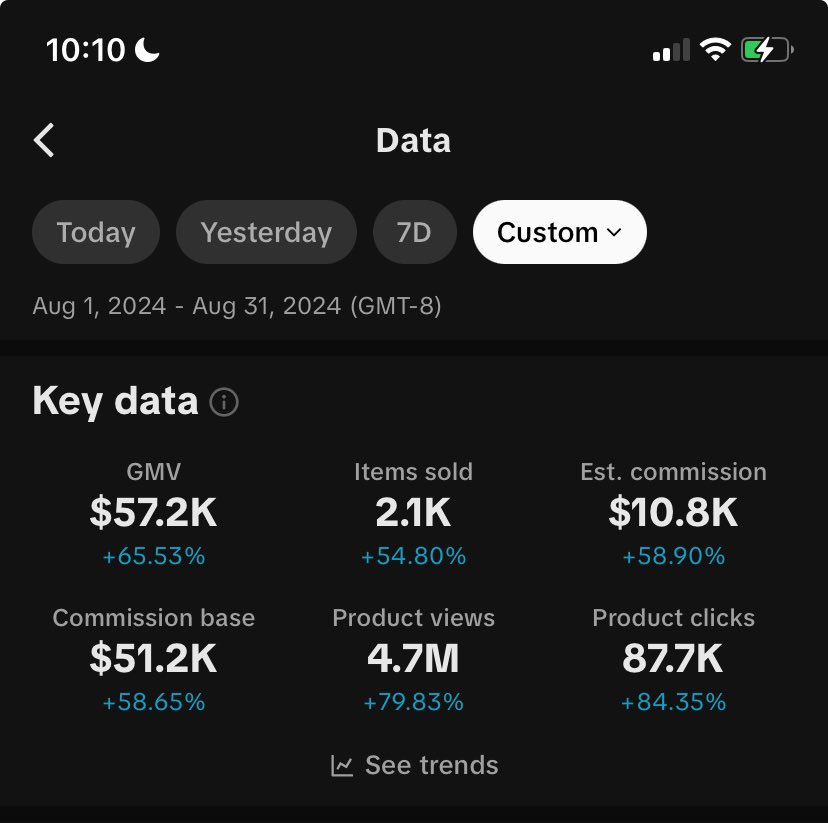
🚨 consumer app on the rise alert 🚨 Breezi got 6k waitlist signups from 550k views. their secret formula ⬇️ iterating on 3 different video formats: founder-led, product-focused + a trending audio, and green-screening on top of the app + talking here's what's doing the… x.com/i/web/status/1…
Learn programming by playing games: Blockchain → cryptozombies.io SQL → mystery.knightlab.com JavaScript → warriorjs.com Go → codingame.com Python → codedex.io CSS → cssbattle.dev Java → tynker.com Git → ohmygit.org
This guy bought land for $450/acre and built a “cinematic off-grid sanctuary” renting unique stays. Now Disney’s paying him big bucks to film there. Here’s what’s going on👇 pic.x.com/KAOVRpV7vd
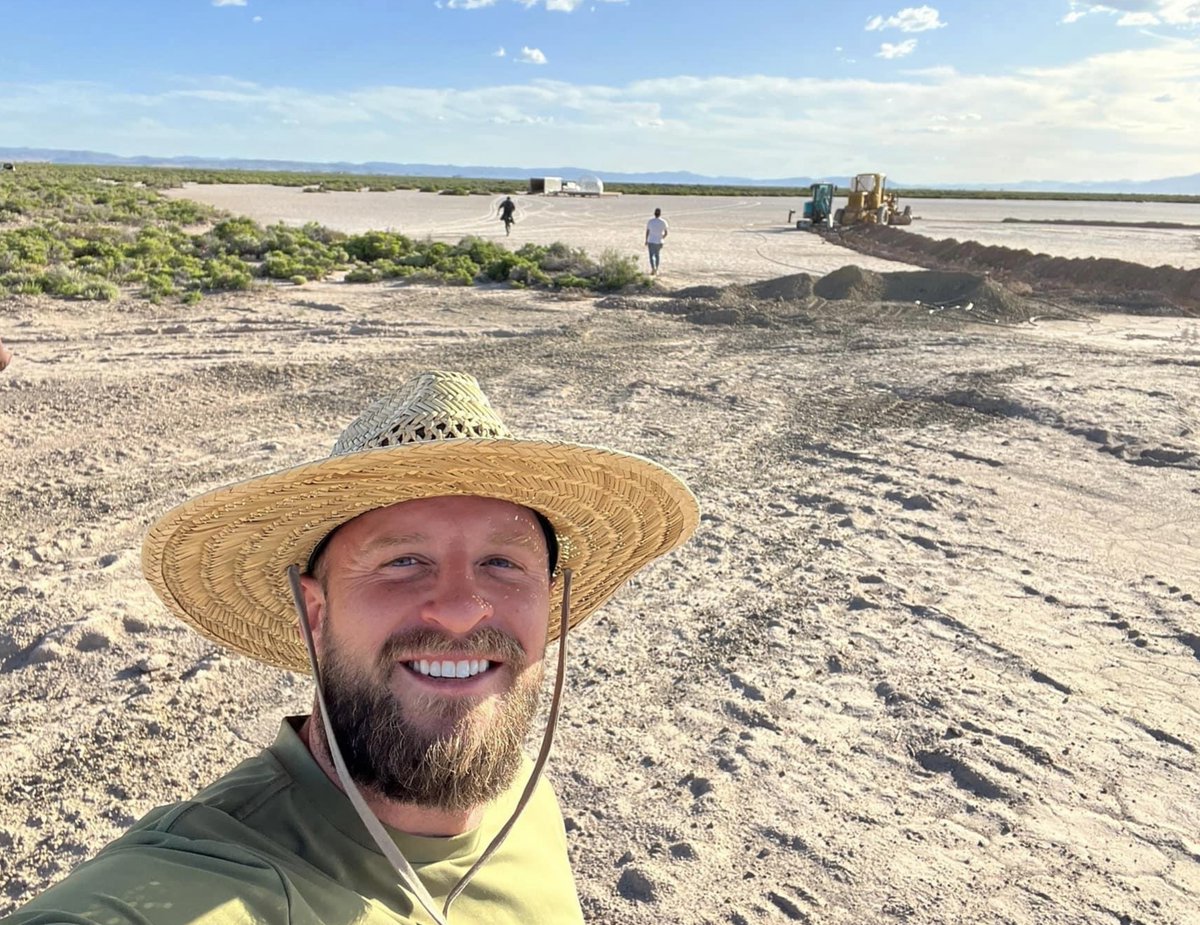